Summary
Background
Socioeconomic conditions affect the dynamics of the Covid-19 pandemic. We analysed the association between area-level socioeconomic deprivation, proportion of non-nationals, and incidence of Covid-19 infections in Germany.
Methods
Using linked nationally representative data at the level of 401 German districts from three waves of infection (January-2020 to May-2021), we fitted Bayesian spatiotemporal models to assess the association between socioeconomic deprivation, and proportion of non-nationals with Covid-19 incidence, controlling for age, sex, vaccination coverage, settlement structure, and spatial and temporal effects. We estimated risk ratios (RR) and corresponding 95% credible intervals (95% CrI). We further examined the deprivation domains (education, income, occupation), interactions between deprivation, sex and the proportion of non-nationals, and explored potential pathways from deprivation to Covid-19 incidence.
Findings
Covid-19 incidence risk was 15% higher (RR=1·15, 95%-CrI=1·06–1·24) in areas classified with the highest deprivation quintile (Q5) compared to the least deprived areas (Q1). Medium-low (Q2), medium (Q3), and medium-high (Q4) deprived districts showed 6% (1·06, 1·00–1·12), 8% (1·08, 1·01–1·15), and 5% (1·05, 0·98–1·13) higher risk, respectively, compared to the least deprived. Districts with higher proportion of non-nationals showed higher incidence risk compared to districts with lowest proportion, but the association weakened across the three waves. During the first wave, an inverse association was observed with highest incidence risk in least deprived areas (Q1). Deprivation interacted with sex, but not with the proportion of non-nationals.
Interpretation
Socioeconomic deprivation, and proportion of non-nationals are independently associated with the incidence of Covid-19. Regional planning of non-pharmaceutical interventions and vaccination strategies would benefit from consideration of area-level deprivation and non-national residency.
Funding
The study was funded by the German Ministry of Health ( ZMV I 1 – 25 20 COR 410 ).
Evidence before this study
We searched MEDLINE via Pubmed on 4th October 2021 for relevant spatial epidemiological literature including medical subject headings: (“COVID-19″[Mesh] OR “SARS-CoV-2″[Mesh]) AND (“Socioeconomic Factors”[Mesh] OR “area deprivation”[tw] OR “socioeconomic deprivation”[tw] OR “socioeconomic inequalit*”[tw]) AND (“Spatial Analysis”[Mesh] OR “spatiotemporal modelling”[tw] OR “spatial epidemiology”[tw] OR “spatial regression”[tw] OR “geographic*”[tw] OR “spatial”[tw]) AND 2019/12/01:2021/10/04[edat].We identified 186 studies, of which 38 studies examined the influence of either specific socioeconomic factors (26 studies), or area deprivation indices (11) on Covid-19 morbidity (24) or mortality estimates (21) using a spatial analysis approach. Studies were predominantly based on cross-sectional designs (30), and only a very limited number considered spatial and temporal dependencies (4).
Added value of this study
Using longitudinal nationally representative data at district level in Germany, we found a higher risk of infection in socioeconomically deprived areas, and in districts with higher proportion of non-nationals. The pathways from socioeconomic deprivation to Covid-19 incidence are particularly shaped by sociodemographic variations in districts as well as structural differences, such as level of urbanisation and population density. While the social gradient in Covid-19 incidence shifted directions and increased across the three waves to the disadvantage of deprived districts, the strength of association between proportion of non-nationals and Covid-19 incidence decreased over time, which shows the relative importance of migration-related aspects in the early phases of the pandemic.
Implications of all the available evidence
Our findings suggest that area-level socioeconomic deprivation, and proportion of non-nationals are independently associated with Covid-19 incidence, but these are not fix in magnitude and considerably affected by spatiotemporal dynamics. Pandemic control strategies, including national and regional non-pharmaceutical interventions and immunisation strategies could benefit from considering these patterns in order to provide tailored and low-threshold services for disadvantaged or at-risks populations.
Introduction
Socioeconomic inequalities in Covid-19 morbidity and mortality show that less privileged groups are at higher risk for infection, severe course of disease,1, 2, 3 hospitalisation,2 and death.4,5 Such inequalities have also been shown at the area-level with high socioeconomic deprivation areas being at higher risk for Covid-19 infections,6,7 and patients living in most deprived areas showing higher hospitalization rates, admissions to intensive care units, and mortality.8 In Germany, Covid-19 incidence was higher in socioeconomically better-off areas during the first wave of infection, while the pattern changed during the second infection wave towards higher incidence in areas with highest socioeconomic deprivation.9However, the relationship between Covid-19 incidence and socioeconomic factors may interact with demographic (age and sex composition of the population) and geographical characteristics, which determine commuting patterns or population density (e.g., the settlement structure, the degree of urbanization),2,3 in addition to spatial and temporal variations that affect the dynamics of the pandemic.10 Importantly, migration and residential segregation may intersect with socioeconomic factors at area-level, and immigrants may cluster in neighbourhoods with lower socioeconomic status (SES),11, 12, 13 but at the area-level in urban settlement structures with better economic opportunities.14 Racial and ethnic inequalities in Covid-19 morbidity and mortality have been widely reported15, 16, 17 and point to structural inequalities and racism as underlying causes.18 Studies considering the role of migration and Covid-19 are still scarce, and indicate that migrants are at higher risk of infection and death, while mixed evidence exists for hospitalisation.19,20 These patterns in migrant groups may be related to language barriers, travel-related risks and international ties, limited access to public-health information provided by authorities, poor access to the health system, or crowded accommodations and higher occupational risks.19, 20, 21 Not considering the intersection between socioeconomic factors and migration may lead to overestimation of socioeconomic determinants of Covid-19 epidemiology, and underestimate the migration-related factors and vice versa.22Area-level index measures of socioeconomic deprivation have hence become instrumental for researchers and policy makers,23,24 but may hide important patterns and pathways from social exposures to Covid-19 outcomes. So far, studies on socioeconomic inequalities in Covid-19 have not considered the intersections of socioeconomic and migration-related factors, and did also not analyse the different pathways underlying the relation between socioeconomic status and Covid-19. Additionally, the vast majority of studies are based on descriptive or cross-sectional designs, and spatial and temporal dependencies in the distribution of disease have rarely been considered.Using nation-wide data at the district level in Germany from three waves of infection, we aimed to quantify the association between area-level socioeconomic deprivation, the proportion of non-national inhabitants as a proxy measurement for immigrants and Covid-19 incidence, while considering demographic characteristics (age and sex), vaccination coverage, settlement structure, as well as spatial and temporal effects. We further explore the potential pathways from area deprivation to Covid-19 incidence, deprivation domains (income, education, occupation), as well as potential interactions between socioeconomic deprivation, the proportion of non-nationals and sex.
Methods
Study design
We conducted a longitudinal small-area spatiotemporal analysis at the level of 401 districts in Germany (Nomenclature of Territorial Units for Statistics, NUTS 3) from calendar week 02-2020 to 20-2021 to explore the association between Covid-19 incidence, socioeconomic deprivation and non-nationals, considering demographic, vaccination, settlement structure, and spatial and temporal factors. Furthermore, we conducted a stratified analysis according to three infection waves in Germany, and a disaggregated analysis based on the deprivation domains (income, education, occupation). The design resembles a retrospective cohort study with districts as unit of analysis.
Data sources
We linked nationally representative data on district level from five different sources, covering: a) Covid-19 incidence, b) area-level socioeconomic deprivation, c) resident population statistics, d) settlement structure (e.g. rural and urban, district type, area type, and rurality), and e) geographical data.Daily data on notified Covid-19 incidence (01/02/2020 to 05/23/2021) on district level, stratified by sex and age groups (0-4, 5-14, 15-34, 35-59, 60-79, 80+ years), was obtained from the national surveillance system of infectious diseases (Robert Koch-Institute).25 Daily notification data was aggregated into weeks. Cases with unknown sex or age (n = 27,083) were excluded from the analysis. Missing data (on age and sex) was assumed to be missing at random and a complete cases analysis was performed. For the stratified analysis, we grouped the weekly data by waves, defined as the time from an increase in Covid-19 incidence (including the observed temporal peak and the subsequent decrease) until the beginning of the next onset of an increase: wave 1 (02-2020 to 27-2020, January until first week of July), wave 2 (28-2020 to 05-2021, second week of July 2020 until first week in February 2021), and wave 3 (06-2021 to 20-2021, second week of February 2021 until third week of May 2021).The primary exposure of interest was socioeconomic deprivation, defined using the quintiles (Q1 to Q5) of the German Index of Socioeconomic Deprivation (GISD – version from 2021) for 402 districts.24 Based on the index values (ranging from 0 to 76), districts with the lowest deprivation (i.e., highest SES) are assigned to the first quintile (Q1) and districts with the highest deprivation (i.e., lowest SES) to the fifth quintile (Q5). Medium-low (Q2), medium (Q3), and medium-high (Q4) deprivation were assigned to quintiles two to four, respectively. The GISD is an area-level composite index measure on socioeconomic deprivation and combines three equally weighted domains (education, occupation, and income of the district population). Each domain is composed of a number of structural indicators that reflect the underlying district’s status for the given domain (education: school leavers without certificate, employed at place of residence with university degree; occupation: unemployed, gross income and wage, employment quota; income: debtor quota, net household income, tax revenue). The domain-specific indicators are weighted based on a factor analysis rotation.26 Due to an administrative district reform in the federal state Lower Saxony in 2016, two districts were merged into one district. Therefore, following previous approaches to address administrative reforms,12 we calculated population-weighted scores based on the underlying index values and categorised the GISD-measures of the 401 districts into quintiles as defined above.We included the most recent population statistics on district level from 2020 from the system of social reporting in official statistics to calculate expected incidence stratified by age and sex groups (see appendix p16), and the proportion of non-nationals among the population of each district. These were assigned to quintiles NN-Q1 (lowest proportion of non-nationals) to NN-Q5 (highest proportion of non-nationals).27 The proportion of non-nationals was examined as a secondary exposure, and served as a proxy for unmeasured migration-related factors that may affect Covid-19 incidence.District data on settlement structure was taken from a database of the Federal Office for Building and Regional Planning.28 The data includes information on a) rural and urban areas, b) district type (district-free cities, urban districts, rural districts with population concentrations, sparsely populated rural districts), c) area type (urban regions, regions with urbanisation processes, rural regions), and d) rurality (ranging from 0 no rurality to 100 complete rurality). Rurality is defined as the proportion of inhabitants in districts with a population density of less than 150 inhabitants per square kilometre.Daily data on administered vaccination doses was obtained from the Digital Vaccination Monitoring provided by the Robert Koch-Institute on federal states level (n = 16) due to absence of data at district-level.29 Data on the second dose was aggregated into weeks. Weekly cumulative vaccination coverage rates in percent on federal states level were then calculated.Geographical polygon data was obtained from the Federal Agency of Cartography and Geodesy30 to create an adjacency matrix of neighboured districts, and for visualisations.The prepared dataset used for the statistical analysis includes observation on Covid-19 incidence for 401 districts, 72 weeks, 6 age-groups, and sex, i.e. 346,464 observations.
Statistical analysis
We first examined the Covid-19 incidence in Germany using weekly crude incidence rates (CIR) per 100,000 population and corresponding Poisson 95%-confidence intervals (95%-CI) stratified by sex and age-groups (0-4, 5-14, 15-34, 35-59, 60-79, 80+), and GISD quintiles (Q1 to Q5). As an estimate of the incidence over time, we calculated the median CIR and associated interquartile range (IQR) for each stratum within GISD quintiles. We further calculated cumulative incidence rates per 100,000 population for considered categorical factors aggregated on district level for the total period and stratified by infection waves.We then assessed the association between socioeconomic deprivation, non-national residents and Covid-19 incidence both for the whole time period and stratified by infection waves using mixed-effects Bayesian spatiotemporal models fitted by the integrated nested Laplace approximation (INLA) approach.31,32 We used the INLA approach as it provides accurate model results with less computational time and costs compared to a simulation-based Markow-Chain-Monte-Carlo (MCMC) approach. Especially for complex models including spatiotemporal effects and large datasets, both of which apply to our analysis, the MCMC algorithm may be very slow and even computationally infeasible.31 The selection of the model that best fit the count data followed a four-step procedure: four intercept-only models to select best-fit likelihood (1), two spatiotemporal random-effects models to select best-fit spatial and temporal effects (2), five mixed-effect models incrementally adjusted for fixed-effects area deprivation, sex, age-groups, proportion of non-nationals, and vaccination coverage (3), and four further separate models based on the best-fit model from step three to test different variables for district settlement structure (4). The selection procedure (p1-27) and best-fit model specification including information on prior selection and configuration (p27) are described in detail in the supplementary appendix.Finally, we selected the best-fit model (model 6, appendix p12) with the lowest Watanabe-Akaike information criterion (WAIC) and also applied this model configurations to stratified datasets of the three infection waves, and also for examining the deprivation index domains (education, income, occupation). We also conducted a sensitivity analysis on deprivation and proportion of non-nationals, using the deprivation index score and the proportion of non-nationals as a continuous variable. Furthermore, we specified two additional models to explore potential interactions between deprivation (dichotomized as ‘low’ and ‘high’ at the median GISD score), and i) sex, and ii) the proportion of non-nationals (dichotomized as ‘low’ and ‘high’ proportion of non-nationals using the median value as cut-off).Based on the results of the best fitting model specification (appendix p27), we calculated relative risks (RR) and corresponding 95%-credible intervals (95%-CrI) to assess the association of socioeconomic deprivation, proportion of non-nationals, sex, age-groups, vaccination coverage, and settlement structure on Covid-19 incidence.The analysis was conducted with the R-programming language (3.6.3) using the R-INLA package.31,32
Selection of covariates
In the mixed-effects models, we assessed the independent association of the exposures (deprivation and non-nationals) with Covid-19 incidence controlled for fixed-effects sex, age-groups, vaccination coverage, and settlement structure (i.e. area type in the best-fit model), and spatial and temporal random-effects. We further explored the pathways of deprivation, i.e. the direct effect from area deprivation to Covid-19 incidence by calculating absolute and proportional changes in the deprivation risk estimates between the incrementally controlled models. See our conceptual diagram of selected variables in the supplement (p3).We first controlled the exposure deprivation for assumed confounders sex and age-groups, which could influence both area deprivation and Covid-19 incidence through different demographical compositions in the districts. We then adjusted the model for the second exposure ‘proportion of non-nationals’ in districts, which serves as a proxy for unmeasured exposures for vulnerable immigrants. Subsequently, we further controlled the model for the cumulative vaccination rate on federal states level, which is assumed to have a preventative association with the outcome, and also a potential mediated association through both exposures, i.e. the impact of vaccination coverage on Covid-19 incidence may be explained by variations in the levels of deprivation and proportion of non-nationals. Finally, we adjusted the model for district settlement structure, which deals as a potential confounder between the association of the exposures deprivation and non-nationals, and the outcome Covid-19 incidence. Settlement structure informs about differences in Covid-19 distribution depending on population density and commuting, may influence the level of deprivation (e.g. through structural advantages in urbanised districts in education, occupation, and income), and informs about the distribution of non-nationals in districts with different settlement structures. In addition, the settlement structure may further have an impact on vaccination coverage, e.g. through structural advantages in urbanised districts to establish and equip vaccination facilities. All mixed-effects models included spatial and temporal random-effects. Spatial variations (covered by district neighbourhood structure and spatial exchangeability within the models) have a direct impact on the regional distribution of Covid-19 cases, and may also explain regional pattern of deprivation and proportion of non-nationals. Temporal variations (covered by weekly structured dynamics and temporal exchangeability within the model) have an impact on time-depending changes in the incidence and potentially also on vaccination rates. Temporal variations inform spatial changes in the distribution of Covid-19 incidence and vice versa. To avoid Table-2-fallacy,33 we report in the main text only the adjusted fixed-effects of the main exposure variables.
Ethic statement
The study builds on anonymised publicly available data from official authorities. No patient-identifying data was used. The study was approved by the Ethics Committee of Bielefeld University (application No. 2022-122)
Role of the funding source
The funder of the study had no role in study design, data collection, data analysis, data interpretation, writing of the report or the decision to publish. All authors (Sven Rohleder, Diogo Costa, and Kayvan Bozorgmehr) have access to the data in the study and had final responsibility for the decision to submit for publication.
Results
In the study period, a total of 3,625,109 Covid-19 infections were notified in Germany, among them 52% (n = 1,868,291; cumulative incidence per 100,000 population = 4434·68) women and 38% (1,388,097; 4799·93 per 100,000) between 35 and 59 years old. About 26% (929,993; 4266·3 per 100,000) of infections occurred in the lowest (Q1) and 16% (566,642; 4721·41 per 100,000) in the highest socioeconomically deprived areas (Q5). In districts with lowest (NN-Q1) and highest proportion of non-national (NN-Q5), 15% (534,289; 5296·13 per 100,000) and 34% (1,228,731; 4706·69 per 100,000) of cases were notified, respectively (Table 1).Table 1Summary statistics of Covid-19 incidence in Germany by infection waves.
Wave 1 (N = 196,476) | Wave 2 (N = 2,074,385) | Wave 3 (N = 1,354,248) | Total period (N = 3,625,109)* | |||||
---|---|---|---|---|---|---|---|---|
n (%) | Cumulative incidence per 100,000 population | n (%) | Cumulative incidence per 100,000 population | n (%) | Cumulative incidence per 100,000 population | n (%) | Cumulative incidence per 100,000 population | |
Age groups | ||||||||
0-4 | 2547 (1%) | 64·30 | 37,306 (2%) | 941·74 | 51,958 (4%) | 1311·61 | 91,811 (3%) | 2317·65 |
5-14 | 5683 (3%) | 76·49 | 120,216 (6%) | 1618·01 | 134,263 (10%) | 1807·07 | 260,162 (7%) | 3501·56 |
15-34 | 50,999 (26%) | 266·76 | 606,604 (29%) | 3172·97 | 406,822 (30%) | 2127·97 | 1,064,425 (29%) | 5567·70 |
35-59 | 80,897 (41%) | 279·74 | 782,749 (38%) | 2706·68 | 524,451 (39%) | 1813·51 | 1,388,097 (38%) | 4799·93 |
60-79 | 35,241 (18%) | 195·16 | 315,446 (15%) | 1746·92 | 184,354 (13%) | 1020·94 | 535,041 (15%) | 2963·01 |
80+ | 21,109 (11%) | 371·56 | 212,064 (10%) | 3732·78 | 52,400 (4%) | 922·35 | 285,573 (8%) | 5026·69 |
Sex | ||||||||
Female | 101,512 (52%) | 240·95 | 1,101,416 (53%) | 2614·38 | 665,363 (49%) | 1579·34 | 1,868,291 (52%) | 4434·68 |
Male | 94,964 (48%) | 231·41 | 972,969 (47%) | 2370·92 | 688,885 (51%) | 1678·67 | 1,756,818 (48%) | 4280·99 |
Deprivation quintiles (score range in percent) | ||||||||
Q1 (0% to <31·80%) | 67,189 (34%) | 308·23 | 531,004 (26%) | 2435·95 | 331,800 (24%) | 1522·12 | 929,993 (26%) | 4266·3 |
Q2 (>31·80% to <40·09%) | 43,486 (22%) | 259·94 | 416,297 (20%) | 2488·47 | 278,491 (21%) | 1664·71 | 738,274 (20%) | 4413·12 |
Q3 (>40·09% to <46·56%) | 43,695 (22%) | 241·86 | 431,674 (21%) | 2389·43 | 276,691 (20%) | 1531·56 | 752,060 (21%) | 4162·86 |
Q4 (>46·56% to <53·24%) | 26,962 (14%) | 185·03 | 373,988 (18%) | 2566·57 | 237,190 (18%) | 1627·76 | 638,140 (17%) | 4379·36 |
Q5 (>53·24% to 76·02%) | 15,144 (8%) | 126·18 | 321,422 (15%) | 2678·17 | 230,076 (17%) | 1917·05 | 566,642 (16%) | 4721·41 |
Proportion of non-nationals (range in percent) | ||||||||
NN-Q1 (2% to <6%) | 14,600 (7%) | 144·72 | 313,337 (15%) | 3105·95 | 206,352 (15%) | 2045·46 | 534,289 (15%) | 5296·13 |
NN-Q2 (>6% to <9%) | 19,012 (10%) | 180·82 | 202,540 (10%) | 1926·29 | 142,589 (11%) | 1356·11 | 364,141 (10%) | 3463·22 |
NN-Q3 (>9% to <11%) | 33,901 (17%) | 232·64 | 322,001 (15%) | 2209·71 | 222,075 (16%) | 1523·98 | 577,977 (16%) | 3966·34 |
NN-Q4 (>11% to <16%) | 57,274 (29%) | 261·7 | 513,748 (25%) | 2347·41 | 348,949 (26%) | 1594·41 | 919,971 (25%) | 4203·51 |
NN-Q5 (>16% to 37%) | 71,689 (37%) | 274·61 | 722,759 (35%) | 2768·55 | 434,283 (32%) | 1663·53 | 1,228,731 (34%) | 4706·69 |
Area type | ||||||||
Urban regions | 98,555 (50%) | 248·28 | 1,072,511 (52%) | 2701·83 | 640,821 (47%) | 1614·33 | 1,811,887 (50%) | 4564·44 |
Regions with growing urbanisation | 60,395 (31%) | 232·69 | 574,559 (28%) | 2213·67 | 419,661 (31%) | 1616·88 | 1,054,615 (29%) | 4063·24 |
Rural regions | 37,526 (19%) | 214·24 | 427,315 (21%) | 2439·57 | 293,766 (22%) | 1677·13 | 758,607 (21%) | 4330·94 |
Caption: Data are n (%) cases, cumulative incidence rate per 100,000 population. *Total N excluding cases with unknown age and sex (n = 27,083, 0·7 %). Area-level socioeconomic deprivation quintiles: lowest deprivation (Q1), medium-low deprivation (Q2), medium deprivation (Q3), medium-high deprivation (Q4) and highest deprivation (Q5). Quintiles of proportion of non-nationals: lowest proportion (NN-Q1), medium-low proportion (NN-Q2), medium proportion (NN-Q3), medium-high proportion (NN-Q4) and highest proportion (NN-Q5). Area type: Urban regions, regions with growing urbanisation, and rural regions. Wave 1: week 02-2020 to 27-2020, Wave 2: 28-2020 to 05-2021, Wave 3: 06-2021 to 20-2021.
- Open table in a new tab
Crude incidence rate
The median CIR across all weeks in deprivation quintiles Q1 to Q5 were, respectively, Q1 39·87 [IQR: 110·81], Q2 26·42 [120·64], Q3 29·81 [107·22], Q4 22·71 [123·45], and Q5 13·78 [125·07]. During the first wave of the pandemic at calendar week 14-2020 (Figure 1), CIR was highest in least deprived (Q1:59·89 [95%-CI 59·88–59·90]) and lowest in most deprived areas (Q5: 17·49 [17·48–17·50]). At the beginning of the second wave (28-2020) until week 48-2020, the inverse association between CIR and deprivation remained, while CIR increased steeply in all deprivation quintiles thereafter and a positive association was observed in the socioeconomically deprived areas. Comparable CIR trends were also observed in the older population groups 60-79 and 80+, and among 35-59 years old (Figure 2). During the peak phases, especially woman aged 35-59 and 80+ showed higher CIR than men. Highest CIR among woman of the mentioned age-groups were found in week 51-2020 in most deprived areas: 359·67 [359·64-359·69] and 489·37 [489·31-489·42], respectively. See CIR by sex and a map stratified by infection waves in the appendix (pp28-29).
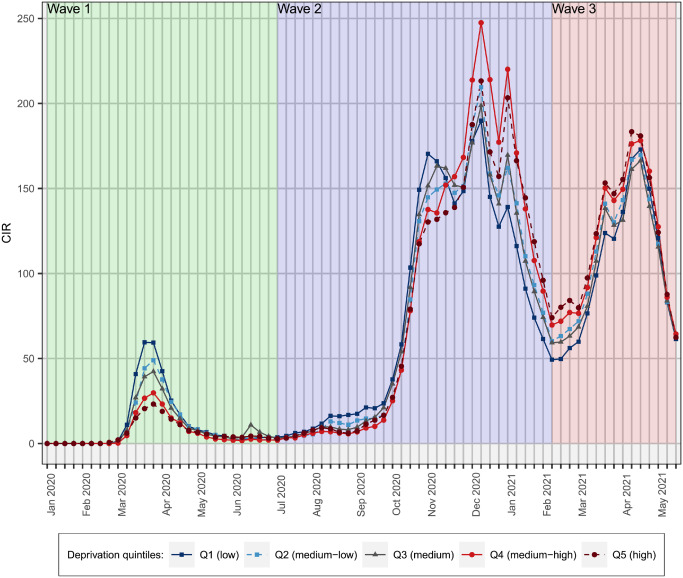
Socioeconomic deprivation, non-nationals and risk of infection
After full adjustment for fixed and random effects, area-level socioeconomic deprivation was positively associated with Covid-19 incidence during the total study period (Table 2 and Figure 3). The risk for Covid-19 incidence in areas with highest socioeconomic deprivation (Q5) was 15% higher than in least deprived areas (Q1). Medium-low (Q2), medium (Q3), and medium-high (Q4) socioeconomically deprived districts had a 6%, 8%, and 5% elevated risk of Covid-19 incidence, respectively, compared to least deprived districts. During the first wave, an inverse association was observed with a 18% and 19% lower risk in most (Q5) and medium-high (Q4) deprived compared to least deprived districts (Q1). In the second wave, the inverse association shifted and showed a gradient from medium-low to highest deprivation, however with highest risk in most deprived districts (Q5) of 11%. In the third wave, the observed risk gradient from wave two sharpened. Compared to least deprived areas (Q1), the RR of Covid-19 infections increased with increasing socioeconomic deprivation from medium-low (Q2: 6%), medium (Q3: 15%), medium-high (Q4: 16%) to most deprived areas (Q5: 29%). For the deprivation domains (Figure 4), we found higher risks in districts with lower education (96%) and income level (14%), but a lower risk in districts with lower occupation level (45% lower risk) during the total period. During the first wave, we found a 87% higher risk of infection in higher income districts. This association shifted during wave two to a 10% higher risk in lower income districts, which increased sharply within the third wave to 112% higher risk of infection in lower income districts. Throughout all infection waves, districts with lower level of education showed higher risk of infection, which increased during the infection waves: 60% (wave one), 77% (wave two), 151% (wave three). Occupation showed lower risk for infections in districts with lower occupational level (i.e. higher risk in districts with higher occupation) throughout all infection waves. Please see estimates for deprivation domains in the supplement (pp15-18).Table 2Relative risks (RR) for Covid-19 infections (fixed-effects) by waves
Wave 1 | Wave 2 | Wave 3 | Total period | |
---|---|---|---|---|
Area-level deprivation quintiles | ||||
Q1 (lowest) | 1 | 1 | 1 | 1 |
Q2 (medium-low) | 1·08 (0·94-1·24) | 1·03 (0·97-1·10) | 1·06 (0·99-1·14) | 1·06 (1·00-1·12) |
Q3 (medium) | 1·00 (0·84-1·17) | 1·03 (0·95-1·11) | 1·15 (1·06-1·25) | 1·08 (1·01-1·15) |
Q4 (medium-high) | 0·81 (0·67-0·97) | 1·02 (0·94-1·12) | 1·16 (1·05-1·27) | 1·05 (0·98-1·13) |
Q5 (highest) | 0·82 (0·66-0·99) | 1·11 (1·01-1·22) | 1·29 (1·16-1·43) | 1·15 (1·06-1·24) |
Proportion of non-nationals quintiles | ||||
NN-Q1 (low) | 1 | 1 | 1 | 1 |
NN-Q2 (medium-low) | 1·19 (1·00-1·42) | 0·92 (0·84-1·00) | 0·88 (0·80 – 0·96) | 0·90 (0·84-0·97) |
NN-Q3 (medium) | 1·20 (0·99-1·44) | 0·98 (0·90-1·07) | 0·92 (0·84 – 1·01) | 0·96 (0·89-1·03) |
NN-Q4 (medium-high) | 1·29 (1·04-1·59) | 1·13 (1·02-1·24) | 1·04 (0·93 – 1·16) | 1·09 (1·00-1·18) |
NN-Q5 (high) | 1·52 (1·21-1·88) | 1·21 (1·09-1·34) | 1·07 (0·95 – 1·20) | 1·16 (1·07-1·26) |
Age groups | ||||
0-4 | 0·41 (0·39-0·43) | 0·65 (0·64-0·66) | 2·96 (2·91-3·01) | 1·05 (1·04-1·07) |
5-14 | 0·34 (0·33-0·35) | 0·74 (0·73-0·75) | 2·62 (2·58-2·65) | 1·01 (1·00-1·02) |
15-34 | 0·70 (0·68-0·73) | 0·97 (0·96-0·98) | 2·02 (1·99-2·05) | 1·10 (1·09-1·11) |
35-59 | 0·80 (0·78-0·83) | 0·85 (0·84-0·86) | 1·95 (1·92-1·97) | 1·04 (1·03-1·05) |
60-79 | 0·87 (0·85-0·90) | 0·84 (0·83-0·85) | 1·76 (1·74-1·79) | 1·01 (1·00-1·02) |
80+ | 1 | 1 | 1 | 1 |
Sex | ||||
Female | 1 | 1 | 1 | 1 |
Male | 1·06 (1·04-1·08) | 1·00 (0·99-1·00) | 1·05 (1·05-1·06) | 1·02 (1·01-1·03) |
Vaccination coverage rate | 0·42 (0·38-0·47) | 1·01 (1·00-1·02) | 0·97 (0·97-0·98) | |
Area type | ||||
Rural regions | 1 | 1 | 1 | 1 |
Urban regions | 1·07 (0·88-1·28) | 1·10 (1·01-1·19) | 0·96 (0·88-1·06) | 1·06 (0·99-1·13) |
Regions with growing urbanisation | 1·06 (0·91-1·24) | 0·95 (0·88-1·02) | 0·95 (0·88-1·03) | 0·99 (0·93-1·04) |
Caption: Relative risks (RR) and corresponding 95% credible intervals (95%-CrI) for Covid-19 infections associated with socioeconomic deprivation (reference: GISD Q1), proportion of non-nationals (reference: non-national Q1), age-groups (reference: 80+), sex (reference: female), cumulative vaccination coverage on federal states level, area type (reference: rural regions). An RR greater than 1 indicates a higher incidence risk. Reference categories refer to a RR equal to 1. Wave 1: week 02-2020 to 27-2020 (January 2020 to June 2020), Wave 2: 28-2020 to 05-2021 (July 2020 to January 2021), Wave 3: 06-2021 to 20-2021 (February 2021 to May 2021), Total period: week 02-2020 to 20-2021 (January 2020 to May 2021).
- Open table in a new tab
The proportion of non-nationals at the district level showed an increasing risk gradient of infection from medium-low (NN-Q2) to highest quintile (NN-Q5) throughout the total study period and infection waves. During the total period, districts with medium-high (NN-Q4) and highest proportion of non-nationals (NN-Q5) showed a 9% and 16% higher risk for Covid-19 incidence compared to districts with lowest proportion of non-nationals (NN-Q1). In the first wave, in districts with higher proportion of non-nationals in quintiles NN-Q4 and NN-Q5 relative to districts with lowest proportion of non-nationals (NN-Q1), a 29% and 52% higher risk for infection was observed, respectively. During the second wave, districts with higher proportion of non-nationals in quintiles NN-Q4 and NN-Q5 showed 13% and 21% higher risks compared to areas with lowest proportion of non-nationals in quintile NN-Q1. In the third wave, we found a similar gradient in the risk estimates with a 7% higher risk in quintile NN-Q5 relative to NN-Q1 (Table 2 and Figure 3).The sensitivity analysis using continuous variables for deprivation and proportion of non-nationals confirmed the findings presented above. From the first to the third wave, the magnitude of the association between area deprivation and risk of infection increased, while the magnitude of the association between the proportion of non-nationals and risk of infection decreased but remained elevated. This shows that, from the first to third wave, the relative importance of area deprivation increased, while the relative importance of the proportion of non-nationals for infection dynamics declined (see supplementary appendix pp19-21 for risk estimates).Please see Table 2 for estimates on sex, age-groups, vaccination coverage, and settlement structure (area type).We did not find a credible interaction between area deprivation and proportion of non-nationals. However, the association with higher proportion of non-nationals tended to be stronger in areas with higher deprivation compared to areas with lower deprivation. The interaction term between area deprivation and sex showed an ordinal, credible interaction: compared to men, incidence among women was higher in areas with higher deprivation. See model estimates, interaction graphs, and a mapping on deprivation and non-national quintiles in the appendix (pp22-26).
Pathways from socioeconomic deprivation to Covid-19 incidence
Absolute and relative changes in risk estimates used to approximate the pathways from socioeconomic deprivation to Covid-19 incidence are visualised in Figure 5. Please see supplementary appendix (p11) for estimate changes for the other considered fixed-effects. After adjusting for sex (Model 2 vs. Model 1), we observed a decrease in the association with Covid-19 incidence in all deprivation quintiles, but increased and flatted again after further controlling for age groups (Model 3 vs. Model 2). Both factors were considered as confounders and showed a considerable impact on the association. When further controlled for the second considered exposure proportion of non-nationals (Model 4 vs. Model 3), the risk estimates in deprivation quintiles Q2 to Q4 increased, while the association in Q5 decreased. This means that not considering non-nationals led to underestimation of deprivation effects in areas with medium-low, medium, and medium-high deprivation, while it led to overestimation of socio-economic factors in areas with highest deprivation. The inclusion of vaccination coverage (Model 5 vs. Model 4) on federal states level marginally decreased the deprivation estimates, however, when controlling for settlement structure i.e. area type (Model 6 vs. Model 5), the risk estimates in all deprivation quintiles increased again (i.e. not considering settlement structure would lead to underestimation of the deprivation effects).
Discussion
Area-level socioeconomic deprivation was positively associated with Covid-19 incidence in Germany during the total study period, as well as in the second and third waves after accounting for the proportion of non-national habitants, and further demographic factors (population size, sex, age), vaccination coverage, area-type), and spatiotemporal factors. We also found that lower education and income level, and higher occupation level in districts particularly affects the Covid-19 pandemic in mutually adjusted models. In the initial phase of the pandemic, we found an inverse association with higher infection risk in least deprived areas. This indicates that “better-off” populations were driving the first transmissions while in the subsequent phases, less privileged groups were at higher risk of infection. A possible explanation may be that those “better-off” or least-deprived were more frequently exposed to international travel in the first stages, while those less privileged, had less possibilities for self-isolation, home-office and worked more frequently in contact-intensive sectors. The magnitude of the adjusted association between deprivation and Covid-19 incidence increased across the three waves.We further found that districts with higher proportion of non-nationals in all infection waves, and within the total study period were at higher risk of Covid-19 incidence, over and above the association of socioeconomic deprivation, age, sex, vaccination, area-type, and spatiotemporal factors. Possible reasons for such higher risk could lie in language barriers to health information, less access to personal protective material and testing infrastructure, more crowded living conditions, higher occupational risks,19 or exposure to international mobility. These reasons could not be further explored in our analysis so that the area-level association should be regarded only as a proxy for other unmeasured factors, which may explain the relationship. The magnitude of the association decreased over the three waves, which may indicate a slow diffusion on relevant health information and pandemic control measures to non-national populations.Information on area-level socioeconomic inequalities of Covid-19 infections are valuable for infectious disease control and policy planning with respect to the initialisation and adaption of sufficient mitigation and containment measures, as well as for an appropriate distribution and prioritisation planning of available vaccine doses on a small-area level.34,35 We show that socioeconomic factors and migration-related factors may operate independently, and need to be taken into account together to design tailored and targeted pandemic control measures.Our findings provide robust evidence that socioeconomically deprived areas are at considerably higher risk for infection than areas with lower socioeconomic deprivation, which is in line with previous explorations.6,7,9 During the first Covid-19 wave in Germany in spring 2020, more cases were reported in socioeconomically advantageous districts than in most deprived areas.36 However, a study on the second wave in Germany in autumn 2020 showed a reversed association, i.e. high socioeconomically deprived areas have notified more cases than less deprived areas.4,37 The associations with deprivation domains, i.e. higher risk in areas with lower education and income level, and higher risk in areas with higher occupation level, indicate that different pathways are at play.38 People with low educational status show lower health literacy and understanding of health information,39 which requires that health systems and authorities communicate in a targeted, non-technical, non-ambiguous and understandable way about the infection risk of Covid-19 and also vaccinations, for example. The income dimension points to material aspects at individual and community level that may affect the possibilities to minimise risk of Covid-19 infections (e.g. using private car versus public transport; provision of testing facilities; staffing of public health services for contact tracing). The association of Covid-19 incidence with higher occupational rates points to the relevance of appropriate and timely implementation of measures that reduce workplace related exposures.Previous research found that, over and above socioeconomic associations, districts with high proportion of non-nationals also showed a higher risk for measles incidence at district level in Germany,40 and for Covid-19 associated deaths and hospitalisations in Sweden.41 In addition, Covid-19 risk varied by ethnicity and specific measures of deprivation (particularly income and employment), but there was a disproportionate risk for ethnic minorities.38 We found that not considering the role of non-nationals in the association between deprivation and Covid-19 leads to both underestimation (in Q2-Q4) and overestimation (in Q5) of socioeconomic deprivation. Based on this, it can be assumed that populations living in socioeconomically disadvantaged areas, and those in areas with high proportion of non-nationals could play a profound role in the spatial and temporal dynamics of the pandemic. This implicates the need to prioritise both socioeconomically disadvantaged populations and districts with higher proportion of non-nationals within national vaccination plans, and to strengthen local low-threshold services in order to reach, inform, and care for these vulnerable populations in regions, which are at higher risk of acquiring infections. Furthermore, we found differential associations of deprivation based on sex, i.e. high deprivation had stronger effects among women compared to men. These interactions may result from social inequalities in payment between woman and men,42 and from a higher proportion of woman working in the health services and care sector. Ignoring the settlement structure leads to an underestimation of the association between deprivation and Covid-19 incidence. For example, urbanised regions compared to rural regions, offer higher capacities of jobs, and educational facilities, thus influencing the income of the district. The interplay of these factors varies depending on the composition of the mentioned factors and can favour the risk of infection by Covid-19 through the individual components as well as in total, e.g. the interplay of several adverse factors in a specific district.An appropriate planning and implementation of non-pharmaceutical interventions (NPI) to contain infection transmission at a small-area level should also consider SES dynamics and the interplay with area-level factors. For example, other measures may be needed in high-deprived-high-migration areas compared to low-deprived-low-migration areas – as opposed to one-size-fits-all approaches that may not be effective depending on the context in which they are implemented. Furthermore, besides discussed SES-drivers for infections, NPI adherence and non-adherence of the population,43 NPI implementability, and also defferential effectiveness of NPIs in the different waves44 may further be important drivers for Covid-19 infections. In addition, social inequalities have been found in the effectiveness of NPIs such as lockdowns showing lower declines of infections in working-class compared to privileged-class individuals.45Our study was based on nationally representative data on Covid-19 incidence, demographic characteristics, and area-level socioeconomic deprivation to model the spatiotemporal association between Covid-19 infections, area deprivation, and the proportion of non-nationals. The small-area analysis at the level of 401 German districts over 72 weeks of disease reporting, and infection waves allowed to estimate the associations of the exposures and covariates, as well as structured and unstructured spatiotemporal dependencies of disease incidence using a sophisticated Bayesian modelling approach. We contributed to a yet small body of evidence on socioeconomic inequalities and Covid-19 morbidity, considering an important intersectional lens, that looks into non-nationals as a vulnerable, at-risk group, and presented a spatiotemporal epidemiological approach that delivers valuable information for district-specific guidance on health political decisions and action planning.Our study was based on aggregated measures of socioeconomic deprivation at district level. The use of an index measure was required, as the notification data on infectious disease does not contain information on individual socioeconomic characteristics of the district population.24 Therefore, conclusions are only valid at the population or district level but not at the individual. Even if individual-level SES of infected cases was available, the study of regional variations by area-level SES would still be meaningful and relevant, as the risk of infection for individuals with high SES may be different in districts with lower area-level SES, and vice versa. We used the German Index for Socioeconomic Deprivation (GISD) from 2021 including updated data until 2017. However, index measures of area deprivation generally show high stability with respect to health inequalities, and vary only marginally over time.46 In addition, the conceptualisation of the GISD is based on international literature and reflects the multidimensionality of area deprivation. However, the GISD remains limited to its domains and indicators included, and also to the usage of mainly process-produced data (as census data in Germany are only available in irregular intervals) to generate the index.24 While notified cases included information on age and sex, and allowed for stratified analysis, an analysis by migration background or nationality was not possible. Instead, we used the proportion of non-national residents as area-level factor to assess associations with overall incidence rates. The risk of spurious associations cannot be completely ruled out. Further, the proportion of non-national residents should be regarded as proxy measure for unmeasured exposures that may have put immigrant groups at higher risk. Data on ethnicity is unfortunately not available in the current district population statistics for Germany. We used the definition of age-groups provided by the RKI. Possible within-heterogeneity of infections due to the composition of groups cannot be excluded, and potential residual confounding could be present in the age-adjusted models, which should be examined in further research using more detailed age-groups exposed to Covid-19.47 Unfortunately, vaccination data was not available on a daily/weekly-basis at the district level in Germany at the time of the underlying study. We therefore used the federal states level vaccination data. Health-related factors, e.g. proportion of care-need or nursing home population, as well as outbreak situations in hospitals and care facilities,48 may further affect the dynamic of infection, which were not included in our analysis, but would be important to examine in future research. Also, reliable daily/weekly data on positive testing rates or comprehensive data on comorbidities are not publicly available on district level in Germany. Our approach to assess potential pathways of deprivation by calculating differences in deprivation risk estimates between the incrementally controlled models delivered important findings on potential paths from area deprivation to Covid-19 incidence. However, the approach did not rely on a distinct pathway-analysis, and further research is needed to validate our findings, e.g. through a structural equation modelling approach. Furthermore, due to administrative territorial boundaries used as geographical level of observation in the analysis (modifiable area unit problem, MAUP) within-district heterogeneity could be present in the included data. The smallest resolution of reporting data in Germany is only available at the district level. However, many containment measures are enacted at this geographic level, for which the results of our study are still informative. We also addressed the role of spatial infection clusters on district level through district-specific risk estimates and corresponding exceedance probabilities (see appendix pp30-31). These estimates show the amount of variance unexplained by the considered variables, and point to the influence of unmeasured potential confounders, such as “high infection clusters” due to single outbreaks or other unmeasured factors.10 We also addressed temporal variations in the incidence (see appendix p32), and used temporal epidemiology of infections for defining the infection waves. However, major changes in mitigation policies coincide with the incidence, which should be examined in future research.Area-level socioeconomic deprivation, and the proportion of non-nationals in districts are associated with higher risk of Covid-19 incidence. The risk of infection increased with increasing level of area deprivation in the second and third waves of the pandemic in Germany, and showed a positive risk gradient for non-nationals throughout all waves. The magnitude of social gradients over time, however, points to late diffusion of protective measures towards migrant populations, while the relevance of socio-economic factors and the protection of populations in highly deprived district would have required stronger attention as the pandemic unfolded from the first to the third wave to avert the higher risk identified in those districts. Tailored NPIs and prioritisation plans for vaccination are needed, which properly consider socioeconomically disadvantaged populations and non-nationals, and low-threshold social and health care services for disadvantaged vulnerable groups should therefore be strengthened. Using the presented spatial epidemiological approach, the infection risk of Covid-19 depending on societal and spatiotemporal factors can be routinely assessed and provide important information for local strategy making.
Contributors
KB, SR, and DC conceptualised the study and statistical methodology. SR collected, curated and analysed the data, and created figures and maps. SR wrote the first and final draft of the manuscript. DC and KB verified data and validated the findings, reviewed and edited revisions of the manuscript for important intellectual content. KB supervised the study. All authors (SR, DC, KB) have access to the data in the study and had final responsibility for the decision to submit for publication.
Declaration of interests
KB reports grants from Federal Ministry of Health Germany (BMG), Federal Agency for Health Education Germany (BZgA), DFG – German Science Foundation, consulting fees from WHO and IOM (UN Migration Agency), honoraria from Federal Agency for Health Education Germany, payments from the German Centre for Migration and Integration (DeZIM). KB has also a copyright on an electronic medical records software [Refugee Care Manager© (RefCare)], and a role on the German Alliance for Global Health Research. All the other authors declare no competing interests.
Data sharing statement
The data used for this study are freely available from the sources referenced. The linked datasets used and/or analysed during the current study are available from the corresponding author on reasonable request.
Funding
The study was funded by the German Ministry of Health ( ZMV I 1 – 25 20 COR 410 ).
Appendix. Supplementary materials
- Download .pdf (2.23 MB)Help with pdf files
- Download .docx (.01 MB)Help with docx files
Article Info
Publication History
Published: June 13, 2022
Identification
DOI: https://doi.org/10.1016/j.eclinm.2022.101485
Copyright
© 2022 The Authors. Published by Elsevier Ltd.
User License
Creative Commons Attribution – NonCommercial – NoDerivs (CC BY-NC-ND 4.0) | How you can reuse
ScienceDirect
Access this article on ScienceDirect
Figures
- Figure 1Crude incidence rates (CIR) per 100,000 population by deprivation quintiles.
- Figure 2Stratified crude incidence rate (CIR) per 100,000 population by deprivation quintiles, sex and age.
- Figure 3Relative risks (RR) for Covid-19 infections of area deprivation quintiles and non-nationals quintiles by waves.
- Figure 4Relative risks (RR) for Covid-19 infections of area deprivation domains by waves.
- Figure 5Changes in risk estimates of area deprivation between adjusted models.
Tables
- Table 1 Summary statistics of Covid-19 incidence in Germany by infection waves.
- Table 2 Relative risks (RR) for Covid-19 infections (fixed-effects) by waves
Article From: eClinicalMedicine
Author: Sven Rohleder Dr. Diogo Costa Prof Kayvan Bozorgmehr